AI in sales planning - PrABCast research project finally completed
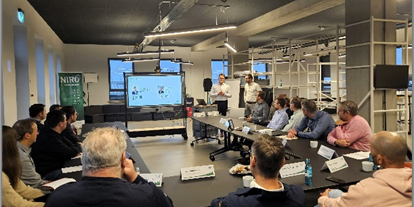
Sales planning is equally relevant for production, sales, purchasing and logistics. Right at the beginning of the workshop, it became clear that artificial intelligence will not replace the often-used ‘crystal ball’ any time soon - but the helpful tool presented, which was developed as part of the project, can improve sales forecasting with the help of analyses and data enrichment.
After a round of introductions, Marius Syberg and Lucas Polley first presented the project approach and the objectives set. The central question was: ‘How can AI be used sensibly in sales forecasting?’
Key success factors for data-based sales forecasts were presented. Data quality is always crucial, as it forms the basis for reliable analyses. In view of the diversity of products and markets, individual modelling approaches are required - and these do not necessarily have to include AI processes. Nevertheless, the integration of external data sources such as market and economic indicators can significantly increase the quality of forecasts. Ultimately, forecasts are always based on analysing past events, as even AI models do not ‘look into the future’ but are based on historical information. In order to recognise developments at an early stage and optimally manage production capacities, it is also essential that the results are comparable.
The comprehensibility and efficiency of AI models also play a major role: models should be easy to explain, understandable and quick to calculate.
In the second part of the event, participants were presented with the project result - a free, easy-to-implement IT tool that tests different forecasting methods, automates sales analyses and integrates external indicators in order to quantify their impact on sales. This tool can be used securely and locally within the company, meaning that it offers the potential to systematically integrate machine learning into sales forecasting in the long term. Use of the tool is free of charge for interested companies.
If you are interested in using the tool or require further information, please contact Marius Syberg and Lucas Polley directly.