Smart Assembly
Development of a novel self-learning gripper system and adaptation of reinforcement learning algorithms as extension of existing lightweight robots for flexible PCB assembly using Through Hole Technology (THT)
Problem and Motivation
Two types of printed circuit board (PCB) assembly technologies are common: the Surface Mount Technology (SMT) and the Through Hole Technology (THT). SMT can be efficiently automated, it is economically more attractive than THT and is continuously gaining importance in PCB assembly. Nevertheless, SMT cannot be applied to produce electronic parts that are exposed to strong mechanical stress or high electrical currents. PCBs assembled using the Through Hole Technology are also more durable under high temperatures. Last but not least, SMT is only economically feasible when large numbers of parts have to be produced, making THT the method of choice for smaller production volumes.
In through-hole assembly, automatic placement is often not possible because the components that have to be installed have widely varying geometric and elastostatic properties and are very sensitive to process deviations in the assembly process. These main obstacles to automating THT assembly can no longer be overcome by conventional programming methods because of the complexity of the problem. Reprogramming and manual adaptation of the control software for new product lines is usually not feasible in practice due to high effort and corresponding costs.
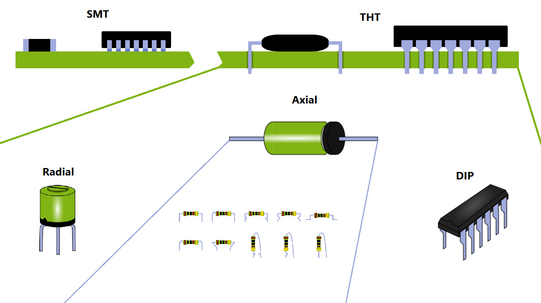
Objective
The aim of the project is to develop a flexible and learning gripper system that can be mounted on existing robots. The gripper system will be suitable for through-hole assembly of different component shapes and will be mountable on several different robots. The reinforcement learning-based control system will replace the programming process with a learning process so that the system can be used flexibly with different component types and product lines without reprogramming.
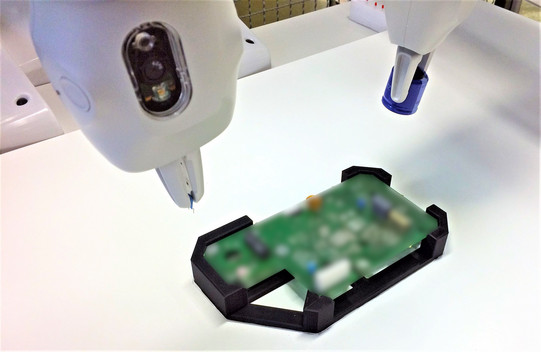
Procedure and Division of Labour
At the beginning of the Smart Assembly research project, suitable reinforcement learning algorithms and training concepts will be chosen that are required for learning the manipulation of THT components based on optical and tactile data. The corresponding sensor technology will be selected taking into account the metrological and economic requirements for the purpose of validation with a real robot system.
In the course of the project, the gripper system will be designed and the associated hardware interfaces and control system will be developed. Special emphasis is placed on the universality and transferability of the developed hardware and software.
Distributed learning independent from robot models and locations will be explored and implemented to accelerate data collection. Thus, a high transferability of anonymized learning experiences between system users and the flexibilization of robotic through-hole assembly for the entire user community will be achieved.
Research-, Development- and Application Partners
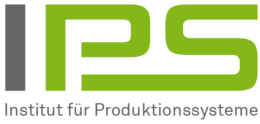
Institut für Produktionssysteme
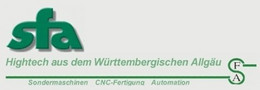
Funding Reference
This Project is supported by the Federal Ministry for Economic Affairs and Climate Action (BMWK) on the basis of a decision by the German Bundestag.