ProData
Cross-Process Chain Detection of Material and Process Anomalies in Imbalanced Data for Engineering Plastic Assemblies (Project processing ist carried out by RIF e.V., Production Systems Department)
Problem
The central problem of the project is to detect and predict anomalies in plastics processing and assembly, especially in scenarios with imbalanced data. The available data from these processes typically has many normal states but few examples of anomalous states, making machine learning model training challenging.
There is a need for effective techniques and methods to efficiently analyze this data and detect process and material anomalies to proactively and predictively monitor and improve process and part quality. Since data evaluation and analysis in the context of plastics processing has been predominantly performed manually and with simple tools such as Excel, there is great potential for improvement through the application of advanced data science methods.
The aim is to create a consistent description of the process states in the injection molding process as well as in the assembly process based on the available material and process data in order to ultimately improve process and component quality.
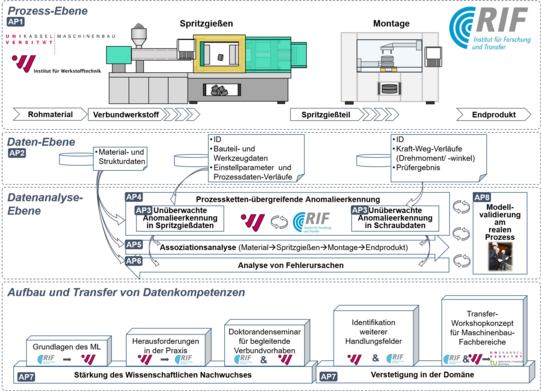
Objective
The main objective of the project is to improve plastics processing and assembly by using artificial intelligence and machine learning. This will be achieved primarily through the early detection and prediction of anomalies.
The first concrete objective is to develop and implement a machine learning algorithm capable of dealing with the unbalanced data typical of such production processes. The algorithm should not only be able to detect anomalies at the process level, but also at the manufactured component level.
The second goal is to apply data science methods to efficiently analyze and predict process and material anomalies. This includes establishing a system for continuous data collection and analysis to support a continuous improvement process.
Another goal is to establish proactive and predictive monitoring and improvement of process and component quality. This should reduce scrap, increase efficiency and save costs.
In addition, we aim to build a prototype system that can test and validate the learned models in the real production environment. This system should be able to provide real-time feedback and detect anomalies before they lead to serious problems.
Finally, we aim to train employees to use the new tools and teach them how to interpret the results. This is to ensure that the system is used effectively and that its benefits can be fully realized.
Overall, the goal of the project is to improve the efficiency and quality of plastics processing and assembly through the use of advanced data science methods and machine learning.
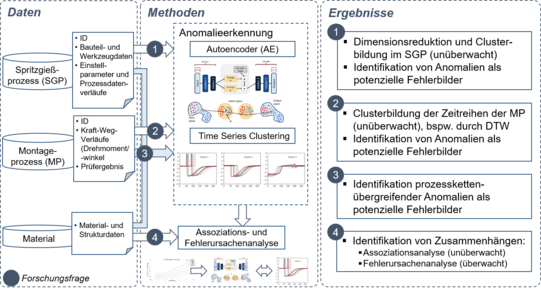
Approach
To achieve the objectives described, a real process chain was established between the University of Kassel, which is equipped with injection molding machines, and the RIF Institut für Forschung und Transfer e.V., which provides an assembly station. The machine, process, material and test data of this process chain will be evaluated across the process chain for the first time.
The specific sub-project of the RIF Institute aims at establishing a common data analysis environment from a data-related perspective. This includes the integration of existing data from the different process steps and their cross-process analysis.
In the first phase, a data science team will merge and clean the collected data to create a robust data basis for model building and analysis. This step is critical to ensure the quality of subsequent analyses and predictions.
Next, machine learning models will be developed and trained to detect anomalies at the process and component levels. These models will be based on the data collected and improved over time through continuous learning.
In the following phase, a prototype system will be developed to implement the models in a real production environment. This system will continuously collect and analyze data to provide real-time feedback and proactively detect anomalies.
In addition, RIF Institute will conduct and coach heterogeneous data analysis of both individual process steps and across process chains. This will ensure that all stakeholders are able to interpret and apply the lessons learned to their work processes.
As the project progresses, we will regularly validate and adjust the models and system to ensure their effectiveness and accuracy. Finally, the successes of the project will be evaluated and further steps will be planned to improve the processes and further implement the learned models.
Research, Development and Application Partners
Funding Reference
The project is funded by the German Federal Ministry of Education and Research (BMBF) and the European Union as part of the funding program "Strengthening Data Competencies for Young Scientists" and is supervised by VDI/VDE Innovation + Technik.
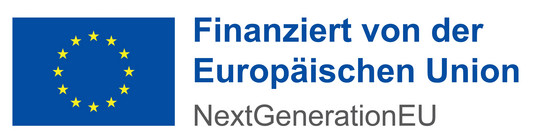
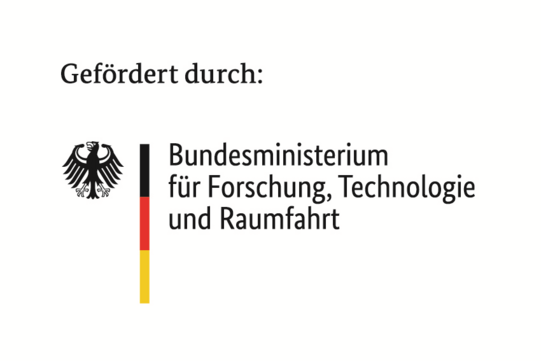