Machine learning solves bottleneck problems in production
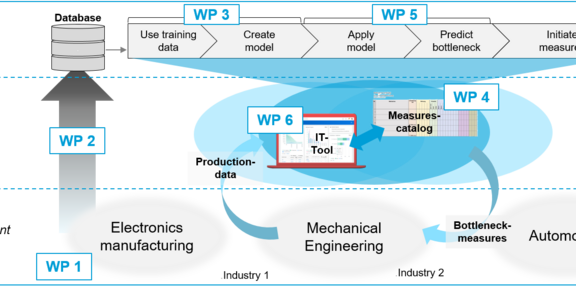
Fluctuating incoming orders, a wide product portfolio and a complex, multi-stage production: these are all general conditions that many manufacturing companies, especially in Germany, have been faced with for more than just a few years. Static and easily controlled bottlenecks are thus a thing of the past in many production environments. Identifying and predicting dynamic bottlenecks is becoming a key competence in improving production efficiency and stability. Often, this is the key approach to further optimize the system capacity. Even though a large number of different approaches and procedures exist in theory and research, their dissemination in practice often shows a clear need to catch up, especially in small and medium-sized enterprises. In industrial practice, problems in the application of theoretical approaches often only become apparent in very specific case studies. For a simplified start, users lack suitable methods and tools to identify for which companies and data situations individual methods are best suited. In addition, there is a lack of practical and efficient software support in the implementation based on the data available in the companies. Since April 2021, the Institute for Research and Transfer (RIF e.V.) has been facing all these challenges together with interested users in the research project "Prediction of dynamic bottlenecks in aligned material flow systems using machine learning methods (PrEPFlow)".
Together with the Project Advisory Commitee (PA), the kick-off of the research project took place on September 7th. The application partners could inform themselves about the current status of the project and the approach of the project. A total of nine companies characterized their application scenarios for bottleneck identification and forecasting. This resulted in exciting scenarios from electronics manufacturing as well as from mechanical engineering and the automotive industry. Based on the scenarios, requirements for a method for bottleneck identification and forecasting were then derived. The derived requirements are, among others, the often unclear data requirements, the transparency of the model results as well as the subsequent mapping of measures to solve the bottleneck scenarios.
By the next PA-Meeting, the derived requirements will be weighted and taken into account accordingly when modeling the bottleneck identification process. In addition, the individual data requirements in the companies will be determined. The next PA-Meeting is scheduled for 12.01.2022. Interested companies are warmly invited to participate in the PA. If you are interested in the research project, please contact Jörn Schwenken (+49 231 755-5187).